Predictable Outputs
The predictability of the model’s output can be controlled using the seed
and temperature
parameters of the Chat API.
Seed
Note
The seed
parameter does not guarantee long-term reproducibility. Under-the-hood updates to the model may invalidate the seed.
The easiest way to force the model into reproducible behavior is by providing a value for the seed
parameter. Specifying the same integer seed
in consecutive requests will result in the same set of tokens being generated by the model. This can be useful for debugging and testing.
Temperature
Sampling from generation models incorporates randomness, so the same prompt may yield different outputs from generation to generation. Temperature is a number used to tune the degree of randomness.
How to pick temperature when sampling
A lower temperature means less randomness; a temperature of 0 will always yield the same output. Lower temperatures (less than 1) are more appropriate when performing tasks that have a “correct” answer, like question answering or summarization. If the model starts repeating itself this is a sign that the temperature may be too low.
High temperature means more randomness and less grounding. This can help the model give more creative outputs, but if you’re using retrieval augmented generation, it can also mean that it doesn’t correctly use the context you provide. If the model starts going off topic, giving nonsensical outputs, or failing to ground properly, this is a sign that the temperature is too high.
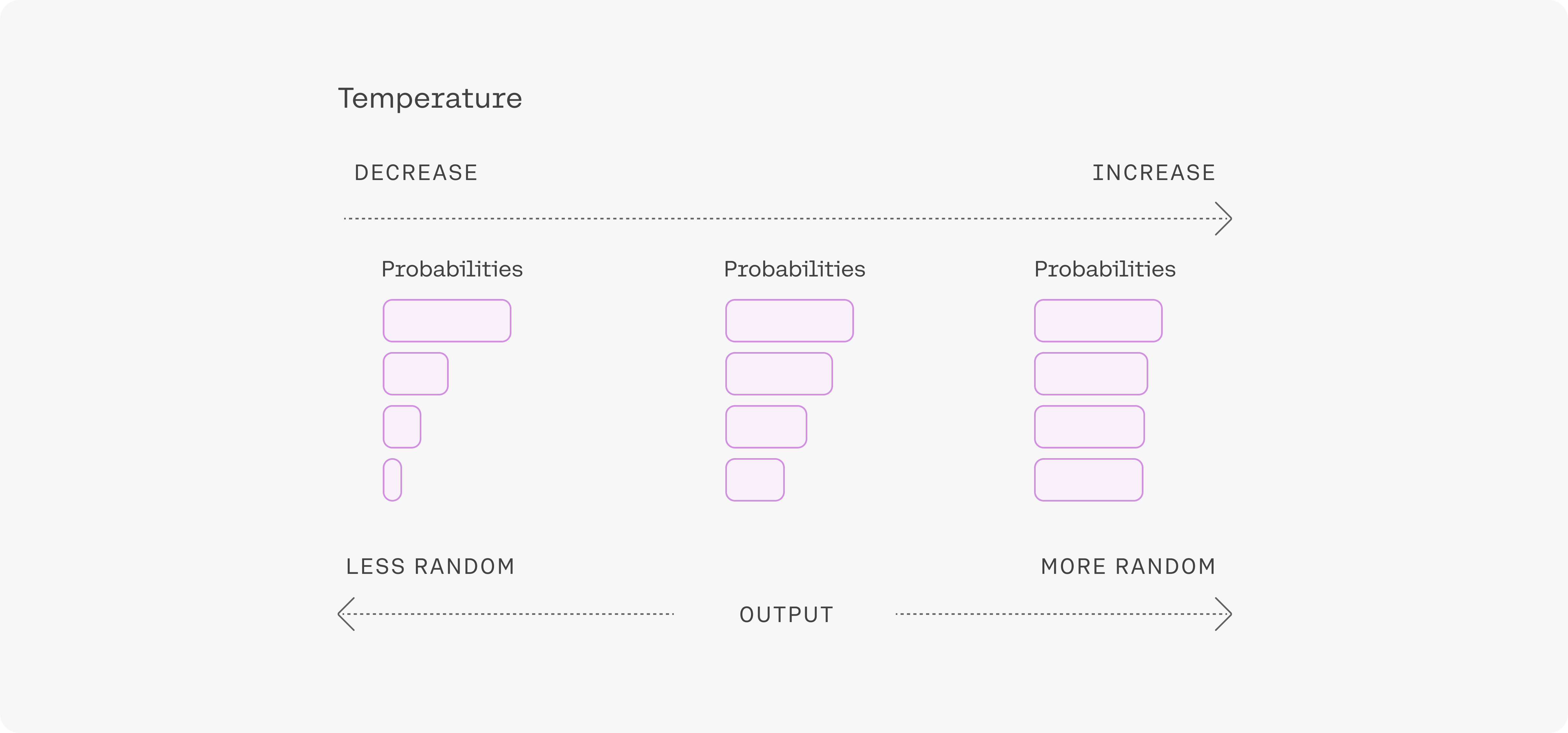
Temperature can be tuned for different problems, but most people will find that a temperature of 1 is a good starting point.
As sequences get longer, the model naturally becomes more confident in its predictions, so you can raise the temperature much higher for long prompts without going off topic. In contrast, using high temperatures on short prompts can lead to outputs being very unstable.